Customer Churn
Why Churn Is Hard to Fight
![]() |
- Churn Is Hard to Prevent
The bad news is that people are (mostly) rational and self-interested, and your customers already know your product. In order to reduce churn long term, and in a reliable way, you have to either improve the value delivered by your product or reduce the cost.
Remembering the last time you churned, what would have prevented you from churning? Better content and features? Maybe. A lower price? Perhaps. How about an improved user interface? Probably not, unless the user interface was terrible to begin with.
And would more frequent email notifications about the product stop you from churning? Again, probably not, unless they contained information that you found valuable. (There’s that value word again!)
To reduce churn, you need to increase value, but doing so is harder than getting people to sign up in the first place.
Because your customers already know what the service is like, promises made by marketing or sales representatives won’t get much traction. As the data person, you may be asked for “silver bullets” to reduce churn, but here is the bad news.
If a silver bullet means a low cost and reliable method, there are no silver bullets to reduce churn!
In the words of the famous startup CEO and venture capitalist Ben Horowitz, “There are no silver bullets for this, only lead bullets.” He was talking about delivering competitive software features in his startup memoir, The Hard Thing About Hard Things (Harper Business, 2014).
But this also applies equally to fighting churn. It means there are usually no quick “once and done” fixes; you continuously have to do the hard work of increasing the value you provide to subscribers. Of course, this doesn't means simple fixes for problems with subscription services never exist.
But these types of issues are usually addressed by people like product managers and content producers. When the service turns to a data person for help in reducing churn, the low-hanging fruit have usually been picked already. If a data person does discover easy fixes, it is a sign that those who created the service have not been doing their jobs well. (It’s possible you will find easy fixes, but you shouldn’t.)
The alternative, of course, is to reduce the cost of the service. But reducing the monetary cost is the nuclear option for a paid service; revenue churn or down sells may be better than a complete and total churn, but it’s still churn.
WARNING Price reduction is a “diamond bullet” against churn: it always works, but you can’t afford it.
- Predicting Churn Doesn’t Work (Well)
Now let’s talk about the usual tool in the data scientists’ toolkit: prediction with a machine learning system.
There are two reasons predicting churn doesn’t work well. First, and most important, predicting churn risk doesn’t help with most churn-reducing interventions. Because there is no such thing as a one-sized-fits-all intervention, churn interventions need to be targeted based on factors other than the likelihood of churn.
This is different from other areas like spam email or fraud detection where yes/no predictions tell you enough to choose an action. If you classify an email as spam, you put it in the spam folder—done! But if you predict a customer is at risk for churn, then what?
To reduce churn, you can run an email campaign to promote the use of a product feature. But a campaign like that should be targeted at users who don’t use the feature, not sent to all users who are churn risks for any reason.
Clogging users’ inboxes with inappropriate content is going to drive them away, not save them! Churn-risk prediction can be a useful variable in choosing customers for one-on-one interventions by Customer SuccessOpens in new window teams, but even then, it is only one variable defining the targets.
This may disappoint you. To reduce churn, it isn’t sufficient to deploy an AI systemOpens in new window that can win a data science competition. If you deliver an analysis that predicts churn without providing more actionable information, the business will not be able to use it easily, if at all. This is one of the most important lessons I had to learn when I started working in this area.
TAKEAWAY A one-size-fits-all churn intervention doesn’t exist, so predicting customers at risk of churn is only a little helpful for reducing churn.
The second reason predicting churn doesn’t work well is that churn is hard to predict with high accuracy, even with the best machine learning. It’s easy to see why, if you recall your behavior the last time you churned: you probably were not taking full advantage of the product, but it took you a long time to cancel because you were too busy or you spent some time researching alternatives.
Perhaps you couldn’t make up your mind, or you forgot. If a predictive system were observing your behavior during that time, it would have flagged you at risk and been wrong during all the time it took you to make up your mind and find the time to cancel. The moment of churn was shaped by too many extraneous factors to be predicted.
Apart from extraneous factors influencing timing, churn is hard to predict because utility or enjoyment is a fundamentally subjective experience.
The likelihood of churn varies from individual to individual, even under the same circumstances. This is especially important for consumer services, where churn is usually hardest to predict.
For business products, customers tend to be rational. But neither the customer nor you have enough information to do a precise cost-benefit analysis on their use of the product.
Finally, churn is normally rare in comparison with intention; it has to be, for any paid subscription that remains in business. Because churn is rare, false positive predictions are common no matter how you make predictions.
Given all these things, churn predictions are inevitably relatively crude. If you worked on project where you predicted churn in the past and found it easy to predict with high accuracy, you might have been predicting churn too late, when it was not actionable.
TAKEAWAY Extraneous factors, subjectivity, incomplete information, and rarity make it hard to predict churn accurately.
- Reducing Churn Is A Team Effort
One of the hardest things about preventing churn is that it is no one’s job, in the sense that no one person or job function can do it alone.
Consider the strategies for churn reduction described earlier: product improvement, engagement campaigns, customer success and support, sales, and pricing.
Those functions span more than half the departments in a typical organization! That means churn reduction is going to suffer from problems of communication and coordination. If left unchecked, there will be a tendency for different teams to come up with uncoordinated approaches to reduce churn.
It would be counterproductive, for example, for the product and marketing teams to decide to focus on driving the use of different features or content. And those approaches may be based on limited or flawed information. Because they aren’t the data experts (that’s you, remember), there’s no guarantee that choices made by independent teams will be properly data driven.
Key Takeaway Churn-reduction efforts are at risk of miscommunication and lack of coordination between the multiple teams involved.
Also, in a typical situation, the data person can’t do anything to reduce churn on their own. Reducing churn depends on actions taken by specialists in different parts of the business, not by a person who is wrangling the data.
These coworkers are diverse, and thus are referred to, by the author, as businesspeople for lack of a better term.
We are not implying that the data person is not part of the business; but data people usually have no direct responsibility for concrete business outcomes (like revenue), whereas the people in those other roles usually do. From the data person’s point of view, the business is the end user of the data analysis results.
Key Takeaway The data person’s goal is to make businesspeople more effective at churn-reducing interventions.
- Weinstein, A. (2002). Customer retention: a usage segmentation and customer value approach. Journal of Targeting, Measurement and Analysis for Marketing, 10(3), 259 – 68.
- Reichheld, F.F. (1996). The loyalty effect: the hidden force behind growth, profits, and lasting value: Boston, MA: Harvard Business School Press.
- Coyles, S. and Gorkey, T. C. (2002). Customer retention is not enough. McKinsey Quarterly, No. 2, 80 – 9.
- Based on Reichheld, F. F. and Sasser, Jr, W.E. (1990). Zero defections: quality comes to services. Harvard Business Review, Sept – Oct., 105 – 11; Reichheld, F.F. (1996). The loyalty effect.Boston, MA: Harvard Business School Press.
- Bolton, R.N. (1998). A dynamic model of the duration of the customer’s relationship with a continuous service provider: the role of satisfaction. Marketing Science, 17(1), 45 – 65.
- Kano, N. (1995). Upsizing the organization by attractive quality creation. In G.H. Kanji (ed.). Total Quality Management: proceedings of the First World Congress. London: Chapman Hall.
- Vivek, S.D., Beatty, S.E. and Morgan, R.M. (2012). Customer engagement: exploring customer relationships beyond purchase. Journal of Marketing Theory and Practice, 20(2), 127 – 45.
- Keaveney, S.M. (1995). Customer switching behavior in service industries: an exploratory study. Journal of Marketing, 59, 71 – 82.
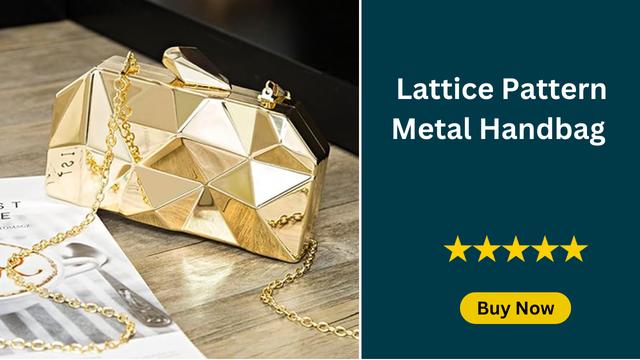